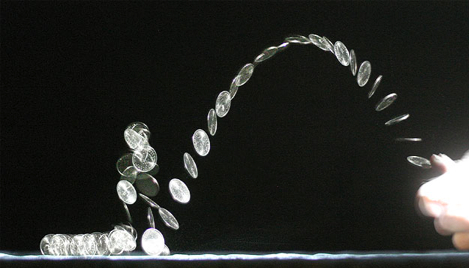
(from http://www.uncommondescent.com/wp-content/uploads/2013/06/coin-flip.jpg)
Sparked by recent events in politics, a lot of debate and controversy has occurred on the Spanish blogosphere around a simple question of probability:
What is the probability that a Yes/No election with 3030 voters results in a tie?
Before suggesting answers, let me make it clear that the main controversy has occurred when trying to answer this question in its barest form, without any additional information besides its simplest formulation above. To make it doubly clear, this is all the information that defines the problem:
1) There are 3030 voters that can vote Yes or No.
2) Yes and No votes are treated as Bernoulli trials.
We model a series of Bernoulli trials with a binomial distribution. It has two parameters, the number of events, and the probability of success for each event:
X ~ Bin(n, p)
Our question is answered by this piece-wise function
P(tie) =
P(X = n/2) if n is even
0 otherwise
All we need to do is plug in the parameters and we’re done. We’ve been given n = 3030 in our problem definition. But wait a minute, what about p? The problem definition states that votes are Bernoulli trials, but we know nothing about p!
In order to create intuition for the situation, let me pose two related questions.
What is the probability of getting 5 heads in 10 trials when tossing a fair coin?
What is the probability of getting 5 heads in 10 trials when tossing a coin where the only thing we know about the coin is that it can land heads or tails?
In the first question we have been given information about the coin we are tossing, which we input into the binomial model. In the second question we know nothing about the coin, and therefore nothing about the second parameter p.
This is precisely the case with our election problem. So how do we proceed? In both cases the answer is the same, we must construct a version of the binomial that allows us to represent this state of information. The beta-binomial probability distribution comes to the rescue. From wikipedia:
In probability theory and statistics, the beta-binomial distribution is a family of discrete probability distributions on a finite support of non-negative integers arising when the probability of success in each of a fixed or known number of Bernoulli trials is either unknown or random.
I hope something rang a bell when you saw the word “unknown” above, this is exactly our situation. What we do, therefore, is to construct a non-informative prior over p that represents our lack of information about said parameter. In the beta-binomial distribution this prior takes the form of a Beta distribution, and the usual choice as non-informative prior is Beta(1, 1), with alpha = beta = 1. You can see how this choice of prior favors no values of p:
Having represented our state of knowledge about p as the choice of prior Beta(1, 1), and given that the parameter n is 3030, we now have all the ingredients to calculate things in a way that is consistent with our problem definition. We do this by using the probability mass function of the beta binomial:
We therefore want (since 3030 is even):
P(X = 1515)
= (3030 choose 1515) * Beta[1515 + 1, 1515 +1] / Beta[1, 1]
= 1/3031
Does that fraction seem funny? That value is precisely one divided by the total number of possible election results. You can see this by considering that results can go from [Yes 0 – 3030 No] all the way up to [Yes 3030 – 0 No]. And in fact, using our beta-binomial model with Beta(1, 1) all these results are given the same probability: 1/3031.
This should come as no surprise, given that as we’ve said repeatedly, the problem definition is such that we know nothing about the election, we have no way to favor one result over the other. You can see this below, the probability for all results is 1/3031 = 0.00033.
The p = 0.5 mistake
In spite of all of the above, most of the people that analyzed our problem got another result, not 1/3031, but instead 0.0145. This corresponds to calculating a binomial distribution with p = 0.5:
X ~ Binomial(3030, 0.5)
P(X=1515)
= (3030 choose 1515) * (0.5^1515) * (0.5^1515)
= 0.01449
How did they get to assuming p = 0.5? Well, it seems that those who went this route did not know about the beta-binomial distribution, and the beta prior that allows us to represent knowledge about p. Without these tools they made an unwarranted assumption: that the lack of information about p is equivalent to 100% certainty that p is 0.5. The source of that confusion is an insidious “coincidence”:
The probability of heads and tails for an unknown coin “happens” to be exactly the same as that for a coin which we know with 100% probability that it is fair.
Let me restate that
P(head for a single coin toss we know nothing about) = 0.5
P(head for a single coin toss we know 100% to be fair) = 0.5
Because the value is the same, it’s easy to jump to the conclusion that a series of coin tosses for a coin that we know nothing about is treated the same way as a series of coin tosses for which we know for sure that the coin is fair! Unfortunately the above coincidence does not reappear:
P(n successes for coin tosses we know nothing about) ≠ P(n successes for coin tosses we know 100% to be fair)
To illustrate that setting p=0.5 (or any other point value) represents zero uncertainty about the value of p, let’s plot a few graphs for priors and probabilities of ties. This will show how our non-informative prior Beta(1, 1) progressively approximates the p=0.5 assumption as we reduce its uncertainty.
- alpha = beta = 1 (non-informative)
probability of tie = 1 / 3031 = 0.00033
- alpha = beta = 10
With Beta(10, 10) the probability of tie increases from 1/3031 to 0.0012:
probability of tie = 0.0012
- alpha = beta = 100
probability of tie = 0.0036
- alpha = beta = 10000
probability of tie = 0.0135
- alpha = beta = 5×10^5
probability of tie = 0.01447
A formal version of the above trend is:
As the variance of our prior tends to zero, the probability of a tie tends to 0.1449 (the value obtained with p = 0.5)
How can we interpret p?
Given the assumption that voter choices are Bernoulli trials, what can be said about the significance of p? We can offer an interpretation, although this won’t change any of our results above.
Having said this, consider that p describes how likely it is that a voter selects Yes or No in an election. If we interpret that a voter chooses Yes or No depending on his/her preferences and the content of the question posed in the election, then p represents the relationship between the election content and the set of voter’s preferences.
Saying that we don’t know anything about the election is like saying that we know nothing about the voter’s preferences and question posed to them. If, for example, we knew that the question was about animal rights, and we also knew that the set of voters were animal activists, then we’d probably have a high value of p. Conversely if we asked gun supporters about gun control, p would be low. And if we asked a generally controversial question to the general population, we’d have p around 0.5.
Unless we have prior information that rules out or penalizes certain combinations of elections questions and preferences, we must use a non-informative prior for p, such as Beta(1,1).
What about using more information?
In most of this post I’ve been talking about an ideal case that is unrealistic. If we do have information about the election beyond its bare description we can incorporate it into our beta prior the same way we’ve done above.
This is precisely what makes bayesian models useful: prior information is made explicit and inferences are principled. I won’t go into the details of this as it would merit a post on its own, only note that using prior information must be done carefully to avoid inconsistencies like the ones described here.